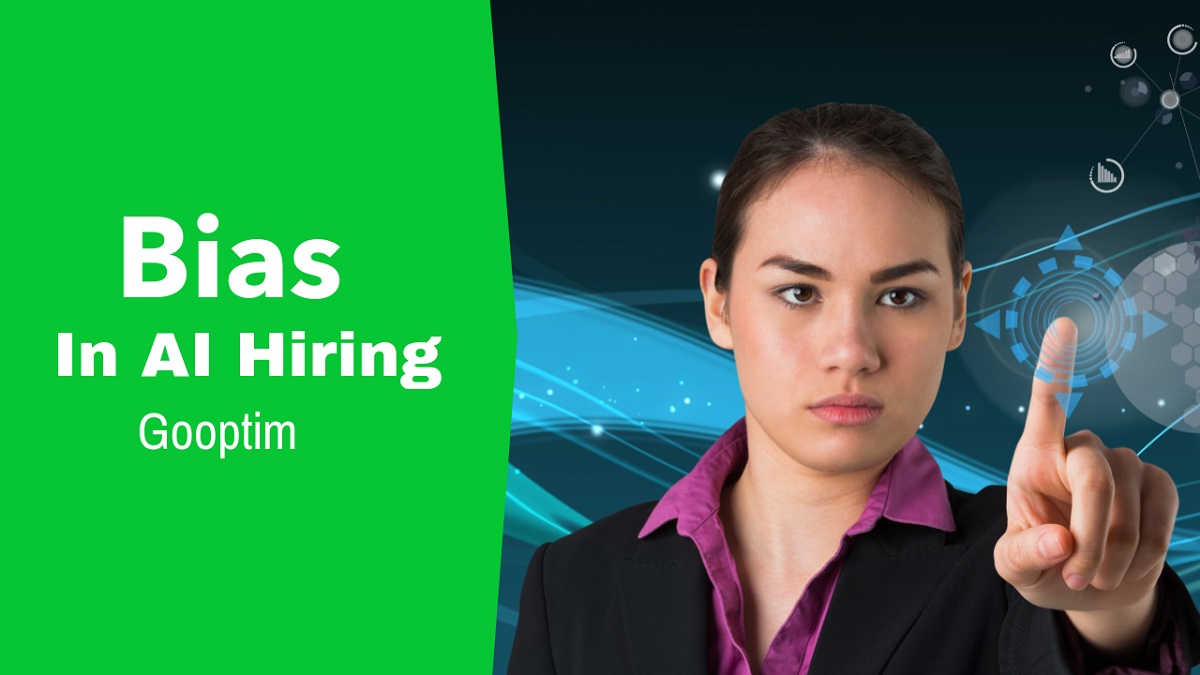
Bias in hiring can also cut down on the number of people who want to work there and put companies at risk of not following government rules.
The company worked hard to get rid of information about protected classes, but after finding bias in hiring recommendations, Amazon decided to end the program anyway.
How does AI bias affect hiring?
AI bias is any way in which AI and data analytics tools can keep bias going or make it worse. This can happen in many ways, but the most common is when the number of people of a certain gender, race, religion, or sexual orientation goes up. Different job offers may be another way that people from different groups earn different amounts of money. Most bias in AI comes from the historical data that is used to teach the algorithms how to work. Even when people try to ignore certain differences, AI gets instructed on biases that are hidden in the data.
There can also be bias in the way the algorithm is made and how people use the results of AI. It can also happen if you use data points like income that are linked to biased results or if you don’t have enough information about successful members of protected classes.
Examples of how AI can be biased when hiring
Bias can creep into the hiring process at the sourcing, screening, selection, and offer stages.
During the sourcing stage, AI could help hiring teams decide where and when to post job ads. Some sites may be better for certain groups than the others, which can make the pool of possible candidates less fair. AI could also suggest different ways to term job offers that would appeal to different groups or turn them off. If a sourcing app notices which recruiters reach out to candidates from certain platforms more frequently than others, it may put more ads on those platforms, which could make recruiter bias worse. Teams may also use coded words, like “ambitious” or “confident leader,” that are more appealing to people from privileged groups.
During the selection stage, resume metrics or chat apps may use certain details to get rid of potential candidates who are indirectly connected to a protected class. They might look for things like gaps between jobs that the algorithm says are signs of productivity or success. Other tools might look at how candidates do in a video interview, which could make bias against certain cultural groups even stronger. For example, there are sports that certain sexes and races tend to play more than others, and there are extracurricular activities that people with more money tend to do more than others.
During the selection stage, AI algorithms might pick some candidates over others based on metrics that are also biased.
After a candidate has been chosen, the company needs to make an offer to hire them. AI algorithms can look at a candidate’s past jobs to figure out what kind of offer they are likely to accept. These tools can make gender, race, and other differences in beginning and career salaries stand out even more.
Four methods to reduce AI bias in hiring
Here are a few of the important ways that organizations can make sure AI isn’t too biased when they recruit and hire people.
1. Keep people informed
Companies don’t just use AI engines on their own; they also involve people in the process. For example, the team in charge of hiring might want to make a way to make sure that the different types of resumes which are fed into the AI engine are balanced. It’s also important to start reviewing recommendations by hand.
2. Exact reasons biased data components
It is important to figure out which parts of the data add bias to the model. When thinking about adding a data point, you should ask yourself if that pattern tends to be more or less common in a protected class or category of employee which has nothing to do with performance. Even though chess players make good programmers, that doesn’t mean that people who don’t play chess can’t also be good programmers.
3. Place a focus on protected groups
Make sure to give more weight to the people from protected groups who may not be represented enough on the staff right now. One client he worked with thought that a degree was a must-have sign of success. After removing this restriction, the client found that employees without degrees not only did a better job but also stayed longer.
4. Calculate progress
Take the time to figure out what success looks like in each role and come up with less biased results, such as more output or less rework. A multivariate approach can help get rid of any bias that may be built into a measurement, such as a performance rating. This new way of looking at things can help you figure out what qualities to look for when hiring new people.
Read more: Recruitment AI Pros and Cons